The Power of Personalization
- Forward
- Personalization and Hyper-Personalization
- Why Does the Enterprise Need Personalization?
- What Can AI Do to Change the Equation?
- Is Generative AI Catalyzing Enterprise Personalization Today?
- Implementation Challenges of Hyper-Personalization in Enterprise
- Personal Data Fusion as a Means for Solving AI Personalization
- How Do I Get on the Personalization Bandwagon?
Forward
It might seem outdated, but the hit 1980s sitcom Cheers tapped into a feeling that resonates with most Americans: a sense of place. A sense of place that exists outside of work and home –sometimes called “the third place” – is reinforced by personalization. The same goes for a member’s club, a volunteering group, a hobby group, or a religious community.
In a business sense, personalization is about making an individual’s experience with a good, service, or tool tailored to their unique preference. We tend to see it in consumer products and services, as it allows companies to differentiate themselves from the competition. It’s why Starbucks (the consumer third place) is adamant about writing the customers’ names on their cups (even if it’s misspelt) and why consumer tech firms go to great expense to personalize their information delivery. But personalization has largely missed one area of society: the workplace. While this seems like a curious omission, we’ll explain why this has been the case.
With the advent of AI and specifically Generative AI making its way into the enterprise, now is an opportune time to explore personalization for knowledge workers, a relatively foreign concept. Will AI be a force for greater enterprise personalization, or is it being applied to the status quo of user interfaces and workflows? Without personalization, can we truly realize productivity gains through AI? These are crucial questions for the future of enterprise work.
In this e-book we’ll make the case for AI as a catalyst for hyper-personalization, how it could enhance work quality and productivity, why we may not be on the right trajectory as of October 2024, and why certain solutions are poised to change that path.
We’ll explore why the enterprise needs to embrace personalization, the barriers to its adoption, and we’ll provide examples of how ModuleQ’s personalizes workflows in wealth management and investment banking through our patented Personal-Data Fusion technology.
Personalization and Hyper-Personalization
Personalization is tailoring the tools, services, and goods workers use to their unique personalities. At the lighter end, this can be achieved by mapping characteristics that describe a person (e.g., age, seniority, geography, affinity). Think about how a product’s front-end may be different if the sign-up process elicits these traits (e.g., a different landing page for people from different countries, different music or film recommendations).
While standard personalization has historically relied on broad characteristics or traits, hyper-personalization goes further by tailoring a user’s experience on categorically unique traits, elicited by their behaviors, interactions, and individual characteristics. Think of a content portal where the content delivery is calibrated to a person’s usage patterns. While we take that for granted as a part of the consumer technology experience, hyper-personalization holds similar promise for the enterprise.
To level set, imagine the first day of work for an investment banking analyst. The young graduate steps into the office for orientation, where they receive a packet of information, including their login and password for their new work terminal. Is any of the software they boot up personalized to them? Is their Workspace data terminal aware of their persona? Chances are it isn’t, in large part because these tools were built to address a huge spectrum of workflows. This anonymizes the user experience to fit the product.
Why Does the Enterprise Need Personalization?
A workhorse piece of software like Microsoft Excel is used by hundreds of millions of people across countless different roles. To function at this scale, it must be generalized. Consequently, we teach workers to conform to its user experience and leverage the functionality they need. But why shouldn’t enterprise software be personalized? Mainly because this “shoehorning” incurs hidden costs.
Over the last 30 years, as enterprise software solutions have matured, we have grown accustomed to conforming our work to these stacks as their use and integration has proliferated. Think of every physical task (e.g., manual book-keeping) that has been replaced by a software-based solution (e.g., accounting software). This led to tremendous productivity gains.
However, the productivity improvement from these workhorse applications and datasets have plateaued, even as our workers gain increasing “day one” familiarity with them through intensive academic training. The solution? More software and datasets!
According to a Statista survey, the average amount of “Software as a Service” solutions deployed within a large company has grown to 130. No single person uses all of them, but the ensemble represents a complex web of tools that require activation, governance, and maintenance costs. As software has proliferated, the most ubiquitous tools and data frames have grown more complex, consolidative, and expansive in their functionality, addressing a wider audience. The result has been a more challenging user experience.
With this spectrum of tools, personalization is ever lacking. Yes, PowerPoint can show you the last dozen or so presentations you’ve worked on when you open the program, but the workflows, calibrations, and information it surfaces are not personalized to you. We’ve trained an entire generation of workers to adapt around this, and we’ve burdened them with the context switching required to cobble together information and tools they find useful and efficient. Personalization is the key to unlocking greater efficiency, better quality work, and more productivity from enterprise applications and datasets.
Personalization
Tailoring digital experiences to individual user needs and preferences.
What Can AI Do to Change the Equation?
AI can push the right information to the right worker, tailored to their workflow. When you step back and think about it, it’s a startling inversion of the status quo. We expect knowledge workers to navigate to disparate systems and elicit files, information, or insight. Why don’t these systems understand the user and simply serve them what is most salient and actionable? This is how AI can change the equation.
Doing so unlocks so much data, tooling, and knowledge at their disposal. With all of the existing spend, there is significant underutilization. This drag is a hidden cost, but simply feels like the water we all swim in.
There are certain catalysts barreling down on the workspace that will make this increasingly apparent. For one, as data providers pivot toward consumption-based models, utilization will be scrutinized. The value of access will be tied with the actual consumption, and personalization is the tendon to connect that data bone to its productive muscle.
There are tremendous social and firm-level gains to be had by addressing this deadweight loss. Personalization can be an overarching approach toward unlocking that value, and AI can be the sherpa for driving personalization. It can do so by curating functionality and user experiences to individuals, especially as their roles and responsibilities evolve over time.
Is Generative AI Catalyzing Enterprise Personalization Today?
Generative AI has certainly spurred discussion around workspace personalization. However, early signs of Gen AI adoption suggest a pattern similar to the adoption of other General Purpose Technologies: new modalities are often wrapped in the same interfaces as their predecessors.
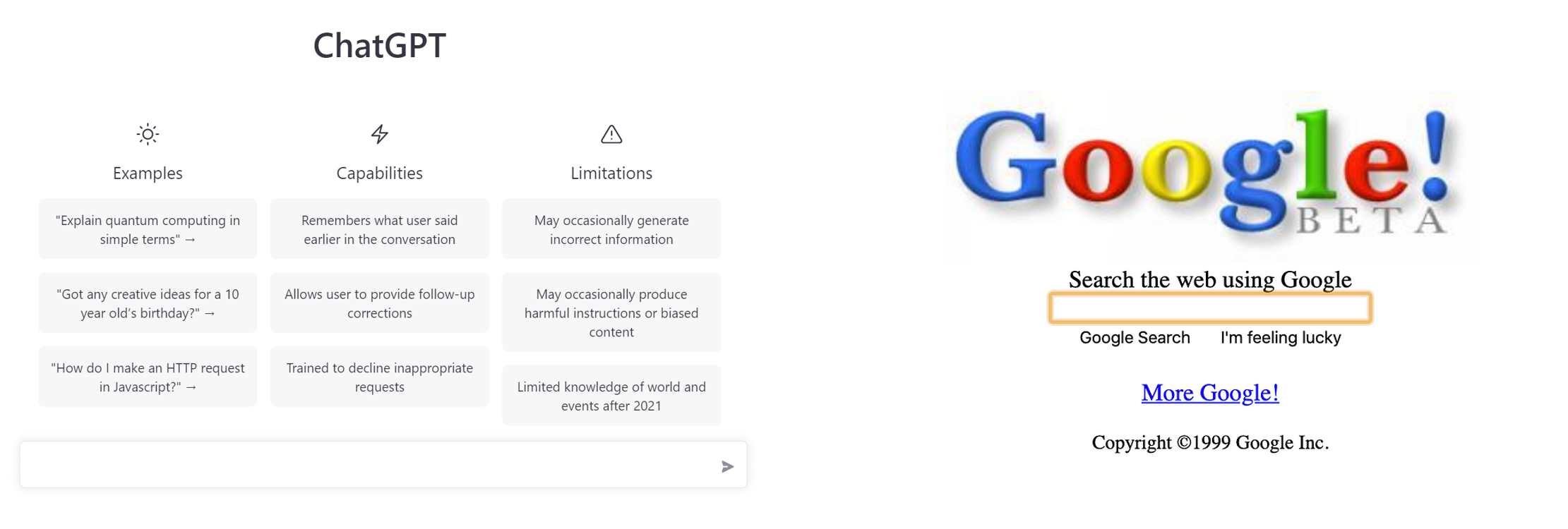
For instance, most people interact with Generative AI through a prompt, much like the search bar we’ve all grown accustomed to since the late 1990s. Even though Generative AI is a new modality, the interface apes the prior version. The tried-and-true interface breeds familiarity for the user and seems logical for the designer but underscores the fact that search is inherently impersonal. This pattern also happened with the first information curation portals appeared on the internet. They were akin to a Dewey Decimal catalog used at a library, the prior home for information storage.
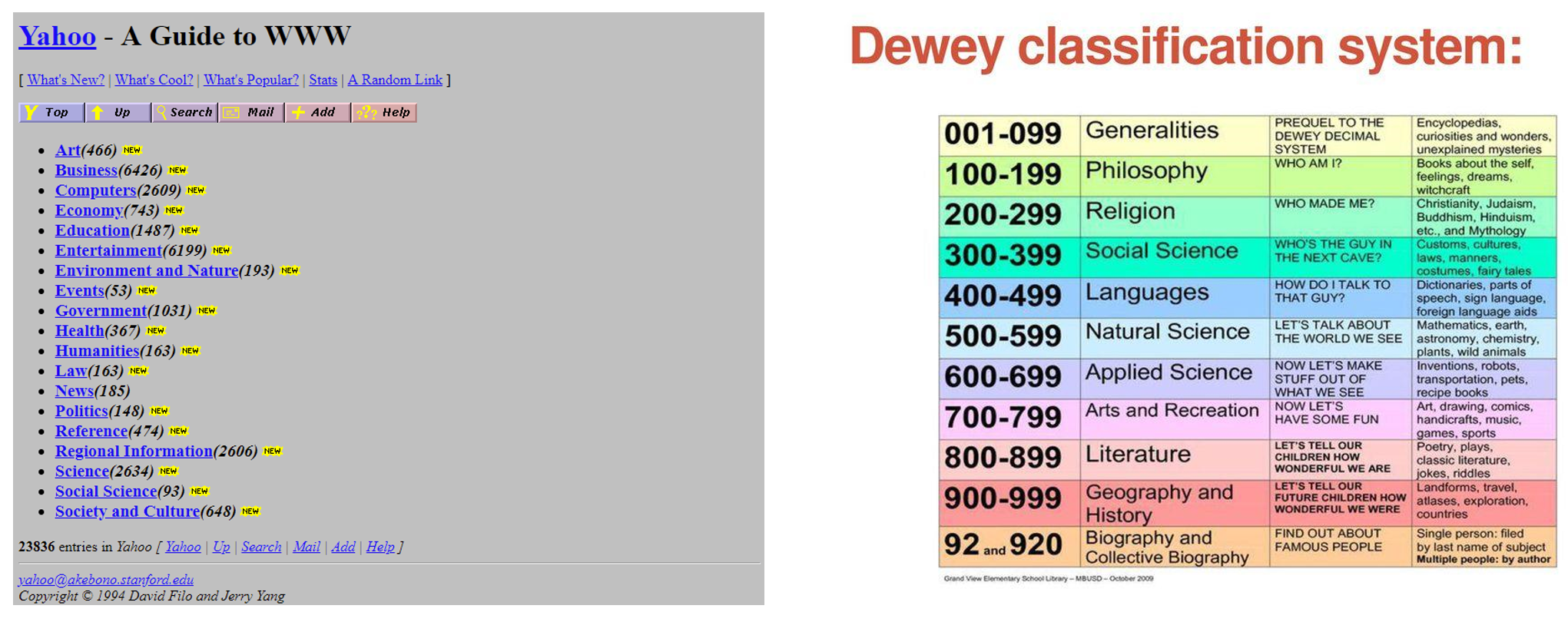
Why is this important? Well, because traditional search is more or less impersonal. It requires the user to personalize the experience through their prompting, rather than the search engine knowing what the user needs. Personalization with prompt-based large language models (LLMs) is a challenge, requiring more “memory,” which currently is a technical and cost-based limitation. Newer versions of LLMs may indeed break this modality, but it remains to be seen how a prompt will fundamentally enable a personalized experience.
Finally, depersonalization also reflects the push by frontier LLM model groups to create true generalized intelligence solutions. With such an approach, personalization is almost an afterthought. We wrote about this in the context of the product versus hardware debate, comparing ChatGPT versus Apple products. The former asks why should I calibrate the product and its output parameters based upon you the person, when it may simply be able to handle all edge cases if it’s smart enough?
At ModuleQ, our information is delivered through Unprompted AI, where information is served to the user rather than being prompted. This necessitates personalization, else we wouldn’t know what to send and to whom! By inverting the interface, we force the adoption of personalization and all of its potential benefits.
Implementation Challenges of Hyper-Personalization in Enterprise
The current instantiations of LLMs aren’t personalized, nor are most enterprise software solutions. Pull dynamics of information retrieval don’t cater to the individual user’s persona, while push dynamics such as Unprompted AI are predicated upon it. What are the other challenges towards implementing hyper-personalization at the enterprise? Let’s examine the consumer space for some clues.
Returning to the example of a social media app, take a step back and think of how many gestures are compatible with an app. It ends up being a handful (swipe, double tap, hold, pinch…what else?). Now compare that to the number of functions or use cases in a spreadsheet program. Easily thousands. You can begin to see why collecting user interaction data from an enterprise software solution is far different than a social media app.
Here lies the rub: consumer technology personalizes in a peculiar fashion. It does so by surveilling one’s activity, using big data algorithms to look for “generally right” patterns, and applying that. It’s a low risk and generally effective consumer interface. If a recommended video misses the mark, it is simply swiped away. While annoying, a consumer doesn’t abandon using the product over a bad recommendation.
It is our belief that true hyper-personalization requires a knowledge graph on users, and most approaches towards knowledge-graph creation comes with some form of attention harvesting. Very few software solutions or companies that are in the business of selling standalone products develop these. Meanwhile, if a recommended article or reference is wrong in an enterprise level, it can lead to stranded adoption. It can’t be “roughly right”, it must almost always be right!
This is a different solution space than what ModuleQ wants to deliver to the enterprise: greater salience of information and thus greater productivity. ModuleQ would rather you interact less with the information that we serve because it is the right information, not needing to hold your attention. Our guiding light is to deliver the right information at the right time to the right person, which is why our approach to personalization is the inverse of most consumer tech.
Privacy is more central to the way we organize our workspace than the way consumers interact with their phones. Data scale is another. Even a large company taps out in the thousands or even tens of thousands of employees. The ability even then to pool them to make valid inference on usage is debatable (how does a junior employee’s use of Excel map with an MD’s? Sales vs Trading?). Social apps are sub-scale when they have hundreds of millions of users. As such, it is a drastically different solution space from a product design standpoint.
Calibration can also be a problem in trying to deliver great personalization for the enterprise worker. Understanding the validity of signals across their specific workflows will be challenging. Knowing which contacts and tasks are important to them is also a challenge, especially given the unique ways different individuals and companies utilize them.
Take for example calendar information. At ModuleQ, we tap into the calendar resources of our investment banking clients to enrich aspects of their daily workflows, such as meeting prep. This delivers a degree of personalization around the type of information we send to our users.
But calendars are used differently by different people. Some supervisors have meetings on their calendars simply for visibility, while others only list meetings which they’ll be attending. This means the validity of the contextual information around a calendar invite can differ and likely needs to be enriched by other contextual clues such as email, user habits, and reinforcement learning.
Personal Data Fusion as a Means for Solving AI Personalization
If we believe that a push medium forces personalization, how do we know what to push? Can we do so without the type of surveillance apparatuses common in consumer tech? To tackle these issues, researchers at ModuleQ have invented Personal Data Fusion (“PDF”), a revolutionary technique backed by military-grade applications, merging key data points within a knowledge worker’s graph with their actual workflows. This requires a foundational understanding of those workflows, instead of simply harvesting their usage patterns across products and portals.
PDF uses your work data—emails, calendar meetings, contacts, etc.—to create a digital twin of you and your work. It does so with privacy controls at the foundational level. That twin is just a shell of yourself, representing common contacts, calendar invites, touch points, but helps power information personalization. You can think of it almost as an assistant who doesn’t know you intimately well but knows enough contextually about how you operate and work to be able to deliver on tasks that shape your day.
Object assessment
Situation assessment
Situation assessment analyzes your relationships with these things and how they are changing over time, so the AI keeps up with you and adapts automatically without any manual updates or configuration.
Impact assessment
Impact assessment predicts the information that will be most useful to you given the specifics of your situation, so the AI can surface crucial insights at just the right moments.
PDF combines the contextual data with information about your persona: what’s your niche or specialty, what’s your seniority, and what type of information do you personally find useful versus noisy. On niche, every role can have slight nuances that a top-down perspective might miss. In Investment Banking, the difference between an equity and debt capital markets banker is huge. The same goes for the sub-categories of industry verticals. Calibrating based upon these specificities can lead to dramatically different information salience. We must adjust our persona-based engineering to account for this.
Additionally, seniority matters. More senior roles need to have more summarization and coordination driven by their data, whereas more junior roles require more ground-level data to help them deliver on work tasks. The result means the type of information that each finds helpful or simply overwhelming often corelates with seniority. Finally on personalization, every bit of information that ModuleQ serves up to a user can be up / down regulated with a click of a like/dislike button. The accumulation of this data allows us to understand the patterns in the data that the user themselves finds useful.
How Do I Get on the Personalization Bandwagon?
There are a few ground level concerns for enterprises looking to adopt personalization to improve workplace productivity. As we mentioned earlier, data integrity and security are key for enterprise deployment. While most consumers are willing to breeze through the security and privacy concerns regarding their data when clicking a Terms of Service checkbox, most enterprises (especially in regulated industries) pay close attention to their data and security.
To that end, having solutions that can sit on-premise greatly improve the ability to apply personalization. AI tools such as PDF remain securely within the security perimeter of the organization. Embedding technology on-prem is thus a key driver of enrichment. ModuleQ has partnered with trusted brands in the financial services space, notably LSEG and Microsoft. This allows us to deliver on data privacy and integrity while still having access to the rich back-end, compute, and market data that can push personalization from a pipe dream to a daily reality.
Becoming Iteratively Better
What gets us really excited about personalization is the ability for improvement to be far faster than a linear trajectory. As more data gets integrated, as more tools connect the dots across that data, and as greater reinforcement fine-tunes that personalization, the productivity boost delivered to the user will only accelerate. And so, without some degree of user feedback, personalization can get its tires stuck on many a pothole.
To fine-tune our process without hampering our users, ModuleQ provides a simple thumbs up/down associated with each of our information delivery cards, ensuring that a user’s preference can help tune and personalize their experience. As more usage patterns emerge through these reinforcement signals, the product’s ability to deliver greater relevance improves, creating a positive reinforcement loop.
The Promise of Hyper-Personalization
A future of hyper personalization at the enterprise is in sight, and it is being driven by advancements in AI technology, improving tools and knowledge graphs of users. It’s important that the industry continues to press on personalization, rather than retreating to the old modality of prompting to access AI. Enterprises must embrace the challenges to reap the rewards of personalization. It must be done in an ethical manner which empowers the worker rather than a surveillance apparatus.
We recognize that it’s a thorny problem. It requires the synthesis of subject matter expertise in the domain with cutting edge technology. It needs a different design ethos that is more “bottoms up” rather than “top down.” It needs to handle security and privacy. If done right, it may be the key to unlocking the next wave of productivity growth at the enterprise level.
At ModuleQ, we will continue to trailblaze in the field of hyper-personalization for knowledge workers. We will continue to adopt a safe and responsible approach; one that does not use surveillance techniques but takes a persona first approach to improving the work experience of knowledge workers. We’re excited to deliver a hyper-personalized work experience, unlocking the next wave of productivity.